Thought in humans and machinesMultidisciplinary Perspectives
17. Oktober 2022
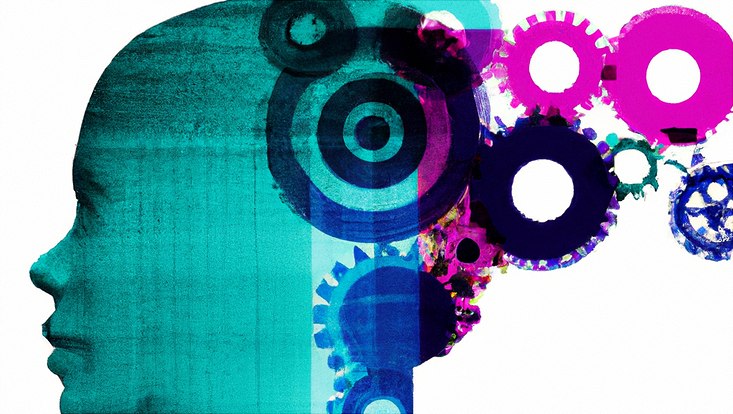
Foto: Regneri/generated with DALL-E (https://labs.openai.com/)
The workshop brings together scholars from different disciplines, such as philosophy, computer science, and neuroscience, that work on (any aspect of) thought in humans and machines (or the machined processes that mirror thought most closely).
The workshop will be in a hybrid format with the option of participating either in person or online.
Talks will be 30 minutes with 30 minutes for discussion – we want to encourage the interdisciplinary exchange!
Time: Monday, November 14th & Tuesday, November 15th, 2022
Place: Warburg Haus, Heillwigstr. 116, 20249 Hamburg, Germany
Organizers:
Michaela Regneri, House of Computing and Data Science, Universität Hamburg
Thomas Kroedel, Department of Philosophy, Universität Hamburg
If you are interested in participating, please email gesa.amann"AT"studium.uni-hamburg.de, indicating whether you’d want to join in person or remotely.
Zoom: https://uni-hamburg.zoom.us/j/67645823330?pwd=NEVMNy95Y1Azbzkrb1cwQTYxTitIdz09
Meeting-ID: 676 4582 3330
Passcode: 32739483
Speakers:
- Anjali Bhat: “Dishbrain and immunoception: Alterity at a biological interface “ (Wellcome Centre for Human Neuroimaging, London)
- Cameron Buckner: Sentimental machines – artificial moral and social cognition in the age of deep learning (Philosophy, University of Houston (Zoom))
- Anne Lauscher: Fairness & Inclusion in modern AI: Cases for Historical Fiction? (Data Science, Universität Hamburg)
- Sara Mann: "Understanding via Exemplification in XAI" (Philosophy, Technical University of Dortmund)
- Dimitri Coelho Mollo: "Models of (Artificial) Intelligence: idealisation and behaviour" (Philosophy, Umeå University)
- Stephanie Nelli: The geometries of flexible knowledge structures in minds and machines (Cognitive Science, Occidental College, Los Angeles)
- Nicholas Shea: How to Solve and Avoid the Frame Problem (Philosophy, School of Advanced Study, London (Zoom))
Abstracts
Anjali Bhat: “Dishbrain and immunoception: Alterity at a biological interface “
Differentiating 'self' from 'other', or alterity, at a biological interface involves a delicate balance of maintaining a boundary while still communicating across it. In this talk, I will introduce two new perspectives on biological alterity that emerge from the action-perception cycle as delineated by the free energy principle (FEP). I will begin by overviewing how the FEP and its corollary, active inference, emerges from perceptual biases well-described in psychology. I then turn to alterity in the immune system and how it may be understood as an inferential process - namely, immunoception. Finally, I will present findings from recent work on an interface between in vitro neuronal networks and in silico computing called DishBrain, which demonstrates this inherent adaptive capacity of biological systems by teaching the neurons to play the arcade game 'Pong'.
Cameron Buckner: Sentimental machines – artificial moral and social cognition in the age of deep learning
While deep learning has demonstrated dramatic success in areas as diverse as perceptual recognition, strategy gameplay, text generation, and predicting the final structure of folding proteins, it has made comparatively less progress on the social and moral aspects of cognition. In this talk, I aim to help deep learning research avoid some less productive avenues to the development of artificial social cognition, avenues focusing on propositional attitude ascription (such as beliefs, desires, and intentions) as the most important target for research. I argue that this target has been so popular in large part because deep learning researchers—whose methodology is a natural fit with philosophical empiricists—have accepted terms of debate set instead by philosophical rationalists. Success in these rationalist frames involves the ability to model particularly tricky propositional attitude ascriptions which could not plausibly be derived from associative learning, such as those involving false beliefs. In the alternative, more empiricist-leaning, sentimentalist tradition—which I will characterize using the work of David Hume, Adam Smith, and Sophie de Grouchy—success instead involves adapting one’s own emotional and affective learning capacities to emulate and coordinate with other agents. Such adaptation—often involving a faculty of empathy/sympathy—facilitates social learning and moral decision-making by helping agents cooperate with one another and solicit help, sanction social defectors, share cultural knowledge through cohesive groups, and eventually achieve the perspective of an "impartial observer" in one of these groups as an aid to normative decision-making.
I make this case by emphasizing and integrating a variety of other research traditions from several disciplines--including sentimentalism in moral philosophy, neuroconstructivism in developmental psychology, affective computing in robotics, and inverse planning in reinforcement learning--to point the way towards the next generation of deep-learning-based agents that can interact with us and one another more successfully by learning to model our moral and social sentiments. I suggest that the impartiality which rationalists have seen as the essence of moral decision-making can be seen as a natural outcome of one of deep learning's greatest strengths--the ability to discover invariances amongst "nuisance" parameters--here understood as controlling for idiosyncrasies amongst the perspectives of differently-situated social agents.
Anne Lauscher: Fairness & Inclusion in modern AI: Cases for Historical Fiction?
The majority of the research efforts in modern AI, e.g., in Natural Language Processing (NLP), are mostly opting for better performance on major benchmarks and downstream tasks. However, it is vital to acknowledge that eventually, these systems will be deployed in a concrete sociotechnical environment, and, thus, their development and their decisions will directly or indirectly affect individuals and more generally, society as a whole.
For instance, NLP systems are prone to encode and amplify unfair stereotypes and often simply fail to adequately represent terms referring to minority groups, which might lead to further discrimination and exclusion of marginalised individuals. In this talk, I will provide an introduction to the training mechanism of modern machine learning-based NLP systems and point to the input data as one potential source of these ethical issues with case studies in conversational AI and machine translation. Our journey leads us to draw from developments in the field of history and take a novel perspective on these problems: considering datasets and models through the lens of historical fiction surfaces their political nature, and affords re-configuring how we view the past, such that marginalized discourses are surfaced.
Sara Mann: "Understanding via Exemplification in XAI"
AI systems that perform image classification tasks are being used to great success in many application contexts. Although achieving high levels of accuracy, such systems are not infallible. The reasons for their failure are not always obvious. The research field of explainable AI (XAI) has therefore developed several approaches aimed at explaining image classifiers. The hope is to bring about understanding, e.g., regarding why certain images are classified as belonging to a specific image class.
In this talk, I suggest to evaluate the suitability of XAI outputs to bring about this understanding through the lens of exemplification. Most of these outputs can be seen as examples of the image class they are intended to explain. By drawing on C. Elgin's work (e.g., 2017) on exemplification, I carve out favorable and unfavorable properties of examples used for explaining image classification, and suggest to focus on generating idealized instances which I call "exemplars". In certain cases, "exemplars" will misrepresent the causes of a certain classification. However, this does not necessarily prevent the acquisition of understanding, but can even facilitate it.
Dimitri Coelho Mollo: "Models of (Artificial) Intelligence: idealisation and behaviour"
In this talk, my aims are twofold. First, I put forward a behavioural characterisation of intelligence that can be applied across the different fields of research concerned with intelligent phenomena, both in biological and artificial systems. I examine how such general characterisation can be applied to AI, showing how it can help assess progress in AI and point toward promising research directions.
Second, I argue that AI research has been both fuelled and hindered by the use of ‘model tasks’, that is, tasks the solution of which are taken to be sufficient for, or at least strongly indicative of intelligence. Reliance on model tasks has led to considerable progress in solving those specific tasks, but it did not lead to theoretical insights about the nature of intelligence in general, and how to build it. This outcome, I argue, is in part due to the failure of recognising the limited scope of model tasks, as well as the abstractions and idealisations of real-world intelligent behaviour that they embody.
Bringing to the fore the limitations tied to such model task choices, as well as the abstractions and idealisations involved in each, I argue, opens the way for a more integrative and plural approach to AI.
Stephanie Nelli: The geometries of flexible knowledge structures in minds and machines
Knowledge structures that are simultaneously flexible and robust are central to the vast repertoire of human behavior. We learn new tasks without forgetting old ones, and rapidly reconfigure our knowledge structures to serve new behavioral goals. To do this, we must minimize interference between tasks to avoid “catastrophic forgetting” of previously learned information. At the same time, this must be balanced with abstraction beyond specific tasks in order to maximize generalization.
What types of neural codes support the ability to protect knowledge structures between relevant relations, but render irrelevant ones plastic? Machine learning models are notoriously bad at multi-task, one/few-shot, and continual learning, and instead perform best when given huge volumes of data in an interleaved fashion. This is strikingly distinct from how humans learn (imagine completing cycles of one question from trigonometry > existentialism > chemistry > medieval history > calculus… and so on). In this example, the temporal autocorrelation inherent in our environments may enable humans to partition or tag relations in a fashion that permits knowledge restructuring (Qian & Aslin., 2014, Collins & Frank 2013).
Interestingly, algorithmic solutions to multi-task, one/few-shot, and continual learning problems, such as altering specific weight subspaces (Zenke et al 2017, Kirkpatrick et al 2017) or clustering representations according to context (Zeng et al. 2019, Bouchacourt et al 2020), implement computations encoding relational certainty during initial learning in a way that helps future knowledge restructuring (submitted: Nelli et al., 2022).
Certainty is not a new idea in cognitive neuroscience (Pouget et al., 2016), but is often reduced to confidence judgments in sequential decision tasks. In recent work, varying the degree of temporal autocorrelation between contexts during initial learning impacts human participant's ability to compose (Flesch et al., 2018) and assemble knowledge (in progress). Importantly, the ability to update knowledge structures is linked to geometrical properties of neural manifolds, as well as meta-cognitive reports in experimental debriefs (in progress). Finally, recent work in fMRI (submitted: Nelli et al., 2022; Okazawa et al., 2021) as well as EEG (Luyckx et al., 2019, in progress) suggests certainty may be explicitly encoded along an axis orthogonal to the decision-relevant feature.
Nicholas Shea: How to Solve and Avoid the Frame Problem
Classical computational systems designed to carry out the kinds of tasks that are characteristic of human intelligence notoriously faced the frame problem – the problem of relevance-based search. Which items of stored information should be retrieved so as to form the basis of inference, reasoning and decision-making? Once a system stores a large database of information, the task of searching through it all to check each item for relevance becomes computationally intractable.
Newer forms of artificial intelligence based on deep neural networks (DNNs) have succeeded in avoiding the frame problem. Inferential dispositions trained into a DNN can effectively take into account a huge amount of information - information encountered during training. But this architecture faces a different and complementary problem, which I here call the ‘if-then’ problem. DNNs are trained to produce the correct outputs in response to a very large number of different inputs. If what they should output depends on the context – on particular variable inputs they have encountered in the past – then the relevant contextual cue can be treated as part of the input. This means that the system has to dedicate resources to being able to deal with a very large number of possible inputs. DNNs have shown that the if-then approach to dealing with context is extremely powerful. It is much more powerful than previously supposed, in particular in large language models where the input can be a whole paragraph of text. That is effectively a very rich context. It is not yet clear where the limits of this if-then style of computation lie, but it does face the constraint that, to deal with contexts that vary, it has to allocate dedicated computational resources to each possible chain of input it might encounter.
That constraint can be overcome by storing explicit memories of situations the system has encountered. When faced with a problem that calls for inference or decision, the system can retrieve relevant memories and draw inferences from them. This solution is now relied on in some hybrid deep learning models. However, as soon as the store of memories becomes large or heterogeneous, the frame problem re-emerges.
How, then, does human cognition overcome the frame problem? I argue that reliance on parallel search in a high dimensional state space, as modelled by DNNs, is an important part of the solution. Human cognition combines that with domain-general computations for which the frame problem really does arise. Various tweaks and kludges help navigate it. But in the human case we can rely on both approaches, taking advantage of the complementary costs and benefits of each. The ability to mediate between these two styles of computational processing amounts to a way of solving the frame problem.